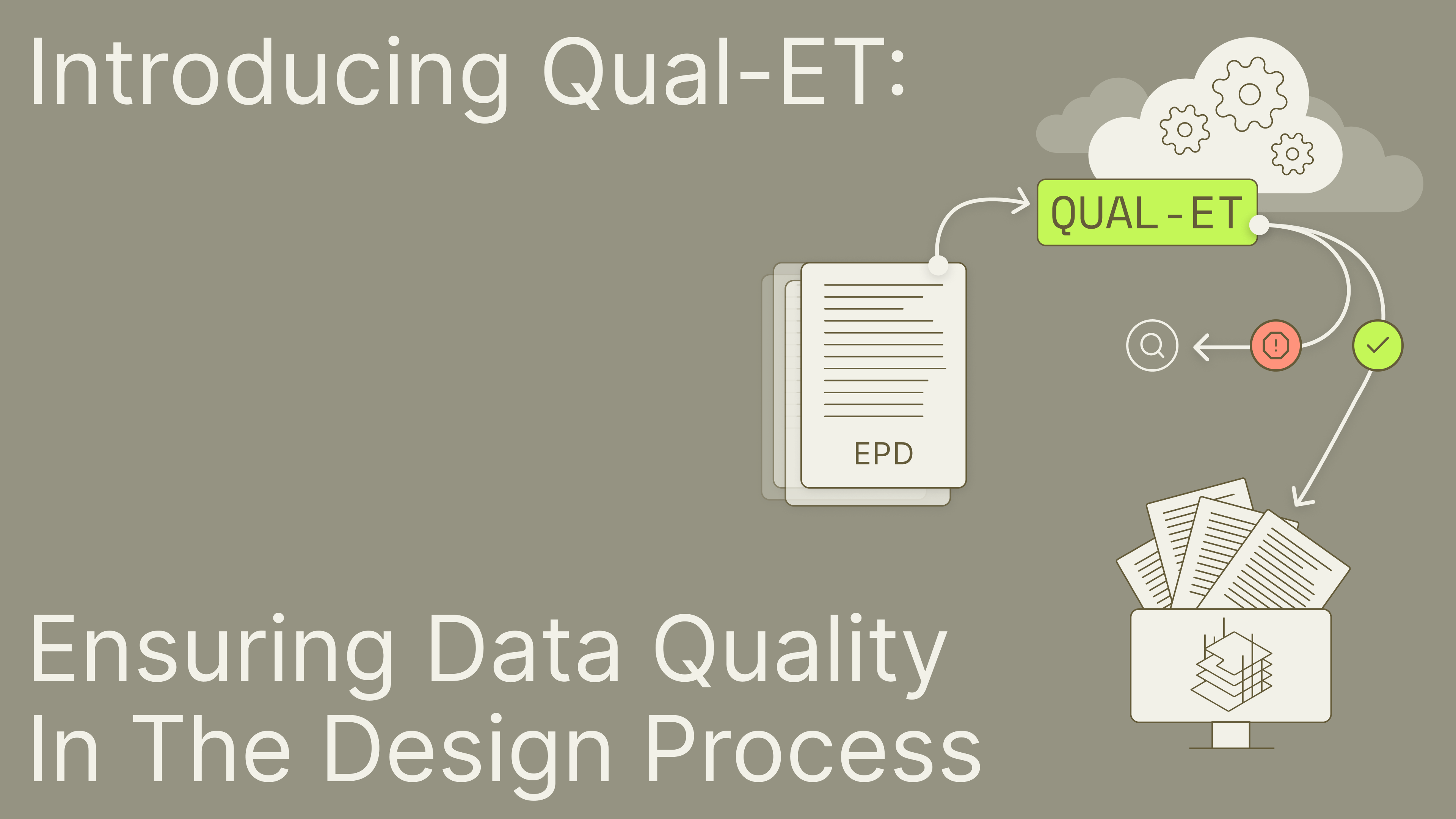
Introduction
Data, in the built environment, is everywhere and with it, all its challenges. From architectural designs to urban planning and infrastructure maintenance, decision-making relies heavily on accurate data.The accuracy and reliability of data can significantly influence the efficacy and sustainability of projects. For example, when comparing the environmental impact of two materials, there should be not much room left for doubt or interpretation. Therefore, the question arises: how do we ensure the quality of our data?
The Built Environment and Data Challenges
Let’s take a look at a practical example of working with Environmental Product Declarations (EPDs). The data within EPDs is often challenging to work with, since inaccuracies, inconsistencies, and outdated information are a not common but still present occurrence. The digitalisation or processing of EPDs are prone to human (and machine) error. Sometimes commas are shifted by one place in either direction, and sometimes numbers simply don’t add up for a particular material. A steel-based product with negative A1-A3 GWP is either the next best thing after sliced bread or a reporting mistake.And the problem only gets magnified when the professionals using the data rely on third-party APIs, as checking the original source gets even more difficult, and data inaccuracies aren’t always so egregious.
Qual-ET, our in-house AI assisted tool, was developed to answer exactly these problems of data accuracy and reliability.. As the name suggests, Qual-ET is used to ensure that datasets in the built environment accurately reflect their expected values.It effectively acts as a gateway between EPD data and our database, automatically flagging parameters that could be out of line.
How Does Qual-ET Work?
Qual-ET cant handle various data types and testing parameters, from GWP to material categories, expiration dates, and more. It can be adapted to fit the specific requirements of different processes and tools, allowing us to set custom parameters for data validation. The boundaries for success/failure in a test are customisable as well. The data that gets flagged is submitted to further automatic checks, until it eventually ends in a log which we ultimately check by hand. When we find errors, such as an expired EPD, or incorrectly transcribed Energy Mix values, we update the database entry to reflect the correct value. If we can’t confirm one at the time, we suspend the material entry, so that no one can use the data by mistake.
Qual-ET is a precious assistant for us as it significantly streamlines the process of capturing and managing the extensive data required for EPDs. This includes information on raw material acquisition, energy use, chemical emissions, and waste generation. By automating the data verification process Qual-ET not only minimises human errors, but also allows our growing but small team to punch above its weight in data procurement.
Conclusion
These accuracy and consistency checks are crucial in our industry where small data discrepancies can lead to significantly skewed decisions, one way or another. Entire teams of architects are often dedicated solely to this task: gathering the right data, double or triple checking the original sources to make sure that the results are accurate… Once again, this means that only the organisations with enough resources can dive into this process. Others, such as smaller or solo firms don’t have this luxury. Bridging the knowledge gap is one of our core missions at revalu, as we think that data-driven decision making should be available to everyone, for the benefit of the environment.
If we want to make sure that bio-based and other innovative buildingsolutions stand a chance to be found and utilised more, we need toensure their data is spot on. Qual-ET is a step in that direction andone we take pride in putting down first.